UX Research Data Analysis is crucial for any research process. Collecting information will have no purpose if your team won’t analyze it properly to gather insights and support decision-making.
Therefore, remember that no matter what kind of research you’re conducting, one of the most critical researcher’s responsibilities is performing data analysis to gather insights and provide recommendations! Keep reading to learn how.
Why should you analyze data?
Making decisions supported only by raw data is a big mistake for any project, including UX Design projects.
At best, skipping analysis will make the design team deliver an incomplete or inefficient solution for users. At the very worst, not analyzing the data can make the design team solve the wrong problem and not even know who their real users are.
Therefore, analyzing data is a fundamental process that drives a project in the right direction, considering users’ needs and gathering insights that help develop better solutions.
A common mistake regarding analysis is jumping to decisions based only on statistical numbers. For example, 60% of users leave the website while on the check-out page.
An impulsive decision regarding this information would possibly be a full-page redesign. But, the problem of users leaving may be related to payment options and methods.
In this case, the redesign would never solve the problem, and that rush decision would probably make the company waste time and money.
This simple example clarifies why analyzing data is important to avoid bad decisions that can harm product development.
Reading tip: UX Research – A Key Role In Product Design
Don’t leave data analysis for last
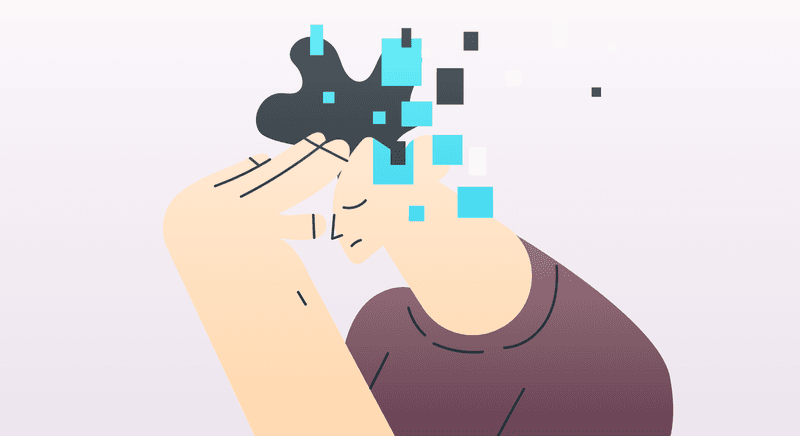
When we talk about UX Research data analysis, a lot of people may think: Okay, we’ll do this work in the end, once we have all data in hand.
However, this strategy is not efficient because, by the end of the process, you will have too much data to analyze, plus all the details and observations that were made during the research.
Therefore, analyze data while it’s fresh out of the oven.
For example, by the end of each interview, take a few minutes to organize ideas and write everything down.
Also, don’t forget to take notes during the interviews; you might have valuable insights that can be lost if you don’t follow your line of thought immediately.
Doing this process as you go makes the final analysis much more organized and easy to make.
How to run analysis during interviews?
Always pay attention and take notes about what users say; the words they choose, their facial expressions, body language, and overall behavior. Every detail counts to fully comprehend their reactions, how they feel, and how they think.
If possible, consider doing the interviews in pairs, so while one person interviews the user, the other observes and takes notes.
These simple steps will make the final analysis much faster once all the insights and observations are documented.
A UX Research process can take weeks or months. So, imagine all the effort to remember small details if you don’t write them right after the interviews are over.
What should you do once the interviews are over?
After the interviews, reserve some time to summarize and organize data and observations.
You can spread the notes over a table and cluster them; look for connections and patterns.
After this, discuss with the team the results and your notes. Then, once you are all together, try to gather everyone’s insights.
This process will provide some important pre-results for the final analysis, which we’ll go through next.
Conducting a UX Research data analysis
Data analysis is the process of turning raw data into relevant information that supports business decisions.
Take a look at a few methods and tools that will help you a lot in this process. There are five fundamental steps to performing a throughout data analysis:

- Defining analysis objectives;
- Organizing data;
- Investigation;
- Clusterization;
- Results and insights identification.
Defining analysis objectives
Analysis objectives are related to the UX Research objectives.
So, keep in mind the reasons why you’ve run a UX Research in the first place, for example:
- What are the user’s needs?
- Which user profile is more likely to use our product?
- On which page is the bounce rate more significant and why?
- What improvements should we make to increase the conversion rate?
- How to make our product more attractive than our competitors’?
These questions help you understand how to conduct the data analysis.
Without a clear objective, the data analysis becomes shallow and useless for the project.
Therefore, the very first step for efficient data analysis is to define the objectives and the answers you are looking for.
Organizing data
In almost every research, you will collect every kind of data, including those that are not relevant to the project.
This way, organizing and filtering information are important to separate useful from useless data.
Moreover, organizing is also about laying out the data so it can be easily found. For example, if a particular webpage has a higher priority, organize data according to which webpage they are about.
This way, you can easily access all data related to the home page or check-out page, for example.
Organizing data is crucial to support efficient data analyses and improve visualization, allowing everyone to better comprehend the numbers and their relevance.
Reading tip: UX Survey – How To Collect Trustful Data
Investigation
The Investigation phase is perhaps where a cognitive effort from researchers is most needed in the whole data analysis process.
The main goal of this phase is to identify words, ideas, or expressions that show up more often in users’ answers and are more likely to be aligned with the analysis objective.
However, an investigation is not about looking for words and their synonymous. Instead, you must know what the words and expressions mean to the users in their own context.
People are different from each other, and groups of people tend to express themselves in a very particular way. So, expressions and words can have different meanings depending on the group of users you are analyzing.
This way, avoid rewriting users’ answers with your own words. Instead, transcribe them exactly the same. Every change or variation in words or expressions can damage the investigation phase and data analysis.
Clusterization
The next step is about creating clusters to label the answers according to what was identified in the investigation phase.
Clusters help the team understand which subjects are a priority and which ones are not.
For example, if a cluster labeled “Interface Performance” was created and you realize that more than half of the users’ answers fit into it, probably the team should prioritize this subject and look for issues regarding interface performance.
Designers organize and analyze large numbers of ideas by categorizing them into clusters.
Results and insights identification
First of all, results and insights are different things.
Results are related to the facts that were found, investigated, and clustered during the analysis process. On the other hand, insights relate to understanding what has caused those results.
This difference exists because users’ answers do not always drive to the root of the problem. Instead, it’s a designer’s job to look deeper and search for insights.
Remember: users are usually unable to identify the root of their problems.
Therefore, the data analysis process pushes the team to see through the results; discuss and find insights, and align findings with the research objectives.
Actually, workshops are a powerful tool to help the team discuss the results and gather insights.
Promote more than one round of discussion to ensure that the insights found are truly the most relevant.
If possible, do a break between one round and another so the team members can clear their minds.
Different research methods, different analysis

The above step-by-step is a general and standard process that works with any research method, qualitative or quantitative.
However, each of these methods has its singularities and you should know how to deal with them accordingly.
Quantitative research data analysis
Quantitative research collects and analyzes numerical data. This method aims to gather quantifiable data and works with statistics and probability, such as:
- the success rate of a specific task;
- how long a user takes to complete a task;
- the bounce rate of a webpage;
- user’s demographic profile.
The quantitative analysis tells a story about how people use and interact with a product while providing specific information about users and their context.
Of course, you can only fully comprehend a user’s story once you analyze both quantitative and qualitative results.
Qualitative research data analysis
Unlike quantitative research, qualitative research works with abstract concepts like human behavior. So you’ll need more time to evaluate their results since they describe ideas, opinions, and experiences.
When you have to analyze qualitative results, ask yourself:
- What do the users like the most about the product?
- What do they like the least?
- Which features are more valuable?
- Why do some users react differently from others?
- Did they have an emotional response? When?
- Are they satisfied with the product? Why?
The qualitative research data analysis provides you with in-depth insights regarding user experience.
Moreover, with the differences between the two methods, it is clear that UX Research must count on quantitative and qualitative results. Complement approaches will provide you with more information, better insights, and outcomes.
Thematic Analysis
As seen above, organizing data and clusterization are two important steps when analyzing data.
At first, these two activities may look simple, but depending on the amount of data, available time, and team expertise, they can become more complex to accomplish.
In this sense, Thematic Analysis is an efficient method that can help you organize data.
Themes and codes
Thematic Analysis works on the identification of themes through codes.
According to Nielsen Norman Group:
- Themes are a description of a belief, practice, need, or another phenomenon that is discovered from the data;
- Codes are words or phrases that act as labels for a segment of text, like a keyword or a hashtag.
In the Thematic Analysis process, codes have a description to help the researcher understand if that’s the segment of text they are looking for.
Codes can either be descriptive or interpretative:
- Descriptive codes describe what the data is about;
- Interpretative codes are an analytical reading of the data, adding the researcher’s interpretive lens to it.
It’s important to understand these definitions to implement Thematic Analysis.
Reading tip: Problem Investigation In UX Design
How does Thematic Analysis work?
The Thematic Analysis has the following workflow:

- Gather all the data;
- Read all the data;
- Code the text;
- Create new codes that encapsulate potential themes;
- Take a break;
- Evaluate the themes.
1) Gather all the data
First of all, you should gather all the raw data regarding the interviews, surveys and any other research method applied.
You must also transcribe audio and video files, so you have in hand all the material in text format. This will make the process easier.
2) Read all the data
The second step of the process is having everyone on the same page. For this, workshops can be a great tool to make the whole team engage with the data you have.
During the workshop session, ask team members to highlight everything they think it’s important.
An interesting technique is giving each member one transcript at a time, instead of making every person read all study entries at once.
This way, when one person finishes reading their transcripts, they can pass them along to another colleague and get a new one. This continues until everyone has read through all transcripts and entries.
3) Code the text
Coding the text means creating categories for the highlighted sections from the previous step. At this stage, it’s essential to have in mind the research objectives, put them up on a wall if necessary.
There are two ways to code the text:
- During the reading phase;
- After the reading phase.
1) Coding while your reading is the traditional approach; as you highlight segments of the data, like sentences or paragraphs, you already code them.
To avoid creating multiple codes for the same type of issue, especially if more people will be coding the text, keep a record of all the codes used and specify what they are. This way, you can refer to this list when coding other sections of text.
2) You will cut up the highlighted sections—physically or digitally—and group all the similar highlighted segments. Then give a code to these clusters.
At the end of this process, you should have data grouped by topics and codes for each topic.
4) Create new codes that encapsulate potential themes
Once you have the first codes in hand, the next step regards finding codes that relate to each other and underlying themes among them.
Then, you will refine and replace the first codes created in the previous phase for ones that better fit the answers and consider more relevant themes.
So at this phase, reflect on the following:
- What is happening in each group?
- Are these codes related? How?
- Are these codes relevant to my research questions?
Analyze the text within each grouping and look for relationships between the data.
5) Take a break
Thematic Analysis involves a huge intellectual load. After a long time reading, creating, and reviewing codes, you should take a break from that.
Clearing the mind is crucial to avoiding mistakes and allows us to conduct analysis more efficiently.
6) Evaluate the themes
The Thematic Analysis’ last step regards reviewing the created codes and themes. At this point, you should open the discussion to more team members, in case you haven’t done it yet.
So, promote an in-depth analysis to ensure that the themes are relevant and if they help the research toward its objectives. Evaluating and reviewing the themes is what helps eliminate bias or wrong interpretations.
Ask yourself:
- Are the themes supported by data?
- Did you find any information that doesn’t support your themes?
- Does everyone agree with the themes you have found in the data after analyzing it separately?
Reading tip: Desk Research – How To Conduct Secondary Research Efficiently
Data analysis and contradictory results
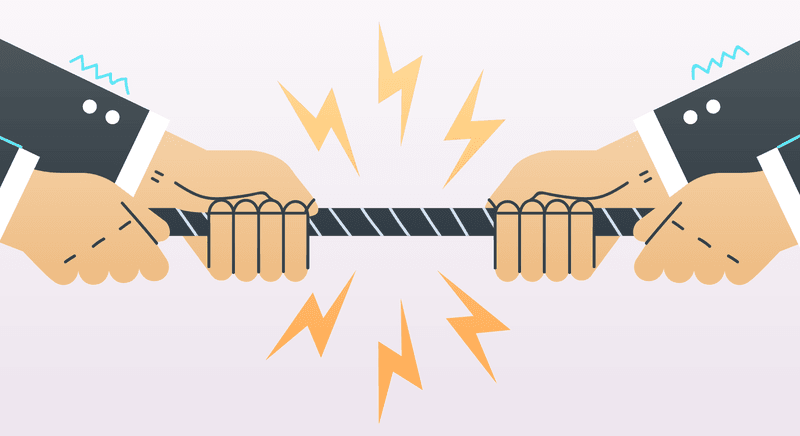
The UX Research process has different approaches to collecting data, considering different points of view in order to gather valid results.
Thus, the usual expectation of the research team is to find that even different methods will point to the same results or insights.
But there are situations where different methods lead the team to contradictory results. In this case, what should you do?
Again, Nielsen Norman Group has an example regarding this situation:
A company developed a new version of a product that their employees use every day to work with. The project team identified that the productivity rate has increased since the launch of that new version.
However, when researchers ran qualitative research to collect users’ feedback, they found that employees were not satisfied with the new product’s version.
This situation sounds contradictory, doesn’t it? A the same time that the productivity rate has increased, users are complaining about the update.
Before closing the case and deciding the results are wrong, the research team has to verify if something went wrong.
Check the methodology
When in contradictory situations, the research team must review the whole process to verify if there is something wrong.
Reviewing the process, the data collected and the results are important to ensure there are no inconsistencies.
You should review the following elements:
- Respondents: Did the same respondents answer both qualitative and quantitative research? Different people may lead to different answers to the same questions;
- Tasks: Are user testing tasks consistent? Every user had the same time to know and interact with the new interface?
- Logistics: Where was the user testing conducted? Does the place interfere with the productivity rate?
- Data analysis: Is there statistical significance? Was the difference in productivity rate considerable?
Everything is okay with the methodology
If the methodology is correct, then maybe new research is required.
In the example above, new research is necessary to understand why users are not satisfied with the product despite the fact that their productivity has increased.
Finding contradictory results is part of the work and happens more often than one might expect.
What are the insights and recommendations?
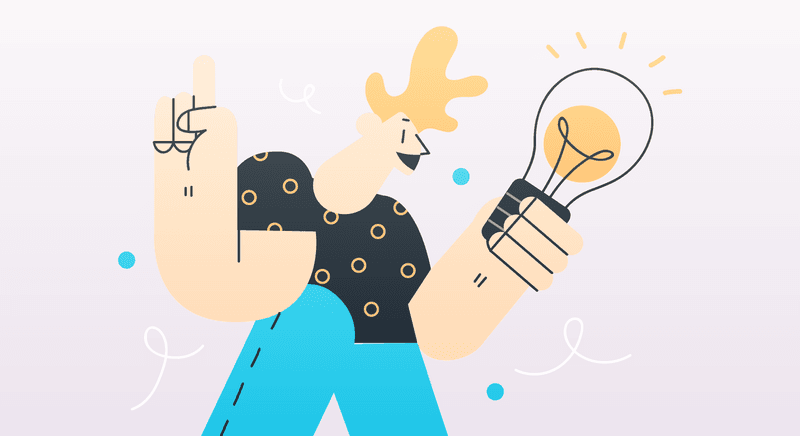
The data analysis final product is a presentation containing insights and recommendations to solve user problems and their needs.
Insight is a description of the research analysis based on the themes and clusters which will support business decisions.
Therefore, insights are different than research results. For example, finding that 60% of users leave the app on the check-out page as we saw earlier, is not an insight.
In this case, the insight could be:
Users feel uncomfortable with the few payment methods available. Moreover, as this information is only communicated on the check-out page, the exit rate becomes higher.
In addition, it is also important that researchers provide recommendations to solve the problems.
Stakeholders may feel frustrated to receive a complete data analysis and insights without any recommendation about the next steps.
Therefore, before presenting the insights, gather the team and discuss recommendations to solve the problems; show them in your presentation and be prepared for questions and feedback.
We hope this article could show you how important UX Research data analysis is and how it can be conducted to provide good insights and recommendations.